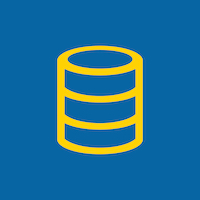
Predict Students' Dropout and Academic Success
Donated on 12/12/2021
A dataset created from a higher education institution (acquired from several disjoint databases) related to students enrolled in different undergraduate degrees, such as agronomy, design, education, nursing, journalism, management, social service, and technologies. The dataset includes information known at the time of student enrollment (academic path, demographics, and social-economic factors) and the students' academic performance at the end of the first and second semesters. The data is used to build classification models to predict students' dropout and academic sucess. The problem is formulated as a three category classification task, in which there is a strong imbalance towards one of the classes.
Dataset Characteristics
Tabular
Subject Area
Social Science
Associated Tasks
Classification
Feature Type
Real, Categorical, Integer
# Instances
4424
# Features
36
Dataset Information
For what purpose was the dataset created?
The dataset was created in a project that aims to contribute to the reduction of academic dropout and failure in higher education, by using machine learning techniques to identify students at risk at an early stage of their academic path, so that strategies to support them can be put into place. The dataset includes information known at the time of student enrollment – academic path, demographics, and social-economic factors. The problem is formulated as a three category classification task (dropout, enrolled, and graduate) at the end of the normal duration of the course.
Who funded the creation of the dataset?
This dataset is supported by program SATDAP - Capacitação da Administração Pública under grant POCI-05-5762-FSE-000191, Portugal.
What do the instances in this dataset represent?
Each instance is a student
Are there recommended data splits?
The dataset was used, in our project, with a data split of 80% for training and 20% for test.
Was there any data preprocessing performed?
We performed a rigorous data preprocessing to handle data from anomalies, unexplainable outliers, and missing values.
Has Missing Values?
No
Introductory Paper
By Mónica V. Martins, Daniel Tolledo, Jorge Machado, Luís M. T. Baptista, and Valentim Realinho. 2021
Published in Trends and Applications in Information Systems and Technologies
Variables Table
Variable Name | Role | Type | Demographic | Description | Units | Missing Values |
---|---|---|---|---|---|---|
Marital Status | Feature | Integer | Marital Status | 1 – single 2 – married 3 – widower 4 – divorced 5 – facto union 6 – legally separated | no | |
Application mode | Feature | Integer | 1 - 1st phase - general contingent 2 - Ordinance No. 612/93 5 - 1st phase - special contingent (Azores Island) 7 - Holders of other higher courses 10 - Ordinance No. 854-B/99 15 - International student (bachelor) 16 - 1st phase - special contingent (Madeira Island) 17 - 2nd phase - general contingent 18 - 3rd phase - general contingent 26 - Ordinance No. 533-A/99, item b2) (Different Plan) 27 - Ordinance No. 533-A/99, item b3 (Other Institution) 39 - Over 23 years old 42 - Transfer 43 - Change of course 44 - Technological specialization diploma holders 51 - Change of institution/course 53 - Short cycle diploma holders 57 - Change of institution/course (International) | no | ||
Application order | Feature | Integer | Application order (between 0 - first choice; and 9 last choice) | no | ||
Course | Feature | Integer | 33 - Biofuel Production Technologies 171 - Animation and Multimedia Design 8014 - Social Service (evening attendance) 9003 - Agronomy 9070 - Communication Design 9085 - Veterinary Nursing 9119 - Informatics Engineering 9130 - Equinculture 9147 - Management 9238 - Social Service 9254 - Tourism 9500 - Nursing 9556 - Oral Hygiene 9670 - Advertising and Marketing Management 9773 - Journalism and Communication 9853 - Basic Education 9991 - Management (evening attendance) | no | ||
Daytime/evening attendance | Feature | Integer | 1 – daytime 0 - evening | no | ||
Previous qualification | Feature | Integer | Education Level | 1 - Secondary education 2 - Higher education - bachelor's degree 3 - Higher education - degree 4 - Higher education - master's 5 - Higher education - doctorate 6 - Frequency of higher education 9 - 12th year of schooling - not completed 10 - 11th year of schooling - not completed 12 - Other - 11th year of schooling 14 - 10th year of schooling 15 - 10th year of schooling - not completed 19 - Basic education 3rd cycle (9th/10th/11th year) or equiv. 38 - Basic education 2nd cycle (6th/7th/8th year) or equiv. 39 - Technological specialization course 40 - Higher education - degree (1st cycle) 42 - Professional higher technical course 43 - Higher education - master (2nd cycle) | no | |
Previous qualification (grade) | Feature | Continuous | Grade of previous qualification (between 0 and 200) | no | ||
Nacionality | Feature | Integer | Nationality | 1 - Portuguese; 2 - German; 6 - Spanish; 11 - Italian; 13 - Dutch; 14 - English; 17 - Lithuanian; 21 - Angolan; 22 - Cape Verdean; 24 - Guinean; 25 - Mozambican; 26 - Santomean; 32 - Turkish; 41 - Brazilian; 62 - Romanian; 100 - Moldova (Republic of); 101 - Mexican; 103 - Ukrainian; 105 - Russian; 108 - Cuban; 109 - Colombian | no | |
Mother's qualification | Feature | Integer | Education Level | 1 - Secondary Education - 12th Year of Schooling or Eq. 2 - Higher Education - Bachelor's Degree 3 - Higher Education - Degree 4 - Higher Education - Master's 5 - Higher Education - Doctorate 6 - Frequency of Higher Education 9 - 12th Year of Schooling - Not Completed 10 - 11th Year of Schooling - Not Completed 11 - 7th Year (Old) 12 - Other - 11th Year of Schooling 14 - 10th Year of Schooling 18 - General commerce course 19 - Basic Education 3rd Cycle (9th/10th/11th Year) or Equiv. 22 - Technical-professional course 26 - 7th year of schooling 27 - 2nd cycle of the general high school course 29 - 9th Year of Schooling - Not Completed 30 - 8th year of schooling 34 - Unknown 35 - Can't read or write 36 - Can read without having a 4th year of schooling 37 - Basic education 1st cycle (4th/5th year) or equiv. 38 - Basic Education 2nd Cycle (6th/7th/8th Year) or Equiv. 39 - Technological specialization course 40 - Higher education - degree (1st cycle) 41 - Specialized higher studies course 42 - Professional higher technical course 43 - Higher Education - Master (2nd cycle) 44 - Higher Education - Doctorate (3rd cycle) | no | |
Father's qualification | Feature | Integer | Education Level | 1 - Secondary Education - 12th Year of Schooling or Eq. 2 - Higher Education - Bachelor's Degree 3 - Higher Education - Degree 4 - Higher Education - Master's 5 - Higher Education - Doctorate 6 - Frequency of Higher Education 9 - 12th Year of Schooling - Not Completed 10 - 11th Year of Schooling - Not Completed 11 - 7th Year (Old) 12 - Other - 11th Year of Schooling 13 - 2nd year complementary high school course 14 - 10th Year of Schooling 18 - General commerce course 19 - Basic Education 3rd Cycle (9th/10th/11th Year) or Equiv. 20 - Complementary High School Course 22 - Technical-professional course 25 - Complementary High School Course - not concluded 26 - 7th year of schooling 27 - 2nd cycle of the general high school course 29 - 9th Year of Schooling - Not Completed 30 - 8th year of schooling 31 - General Course of Administration and Commerce 33 - Supplementary Accounting and Administration 34 - Unknown 35 - Can't read or write 36 - Can read without having a 4th year of schooling 37 - Basic education 1st cycle (4th/5th year) or equiv. 38 - Basic Education 2nd Cycle (6th/7th/8th Year) or Equiv. 39 - Technological specialization course 40 - Higher education - degree (1st cycle) 41 - Specialized higher studies course 42 - Professional higher technical course 43 - Higher Education - Master (2nd cycle) 44 - Higher Education - Doctorate (3rd cycle) | no |
0 to 10 of 37
Dataset Files
File | Size |
---|---|
data.csv | 520.7 KB |
Reviews
There are no reviews for this dataset yet.
pip install ucimlrepo
from ucimlrepo import fetch_ucirepo # fetch dataset predict_students_dropout_and_academic_success = fetch_ucirepo(id=697) # data (as pandas dataframes) X = predict_students_dropout_and_academic_success.data.features y = predict_students_dropout_and_academic_success.data.targets # metadata print(predict_students_dropout_and_academic_success.metadata) # variable information print(predict_students_dropout_and_academic_success.variables)
Realinho, V., Vieira Martins, M., Machado, J., & Baptista, L. (2021). Predict Students' Dropout and Academic Success [Dataset]. UCI Machine Learning Repository. https://doi.org/10.24432/C5MC89.
Creators
Valentim Realinho
vrealinho@
Instituto Politécnico de Portalegre
Mónica Vieira Martins
mvmartins@ipportalegre.pt
Instituto Politécnico de Portalegre
Jorge Machado
jmachado@ipportalegre.pt
Instituto Politécnico de Portalegre
Luís Baptista
lmtb@ipportalegre.pt
Instituto Politécnico de Portalegre
DOI
License
This dataset is licensed under a Creative Commons Attribution 4.0 International (CC BY 4.0) license.
This allows for the sharing and adaptation of the datasets for any purpose, provided that the appropriate credit is given.